AI in healthcare - defensibility, capabilities, and cost reduction
Get Out-Of-Pocket in your email
Looking to hire the best talent in healthcare? Check out the OOP Talent Collective - where vetted candidates are looking for their next gig. Learn more here or check it out yourself.
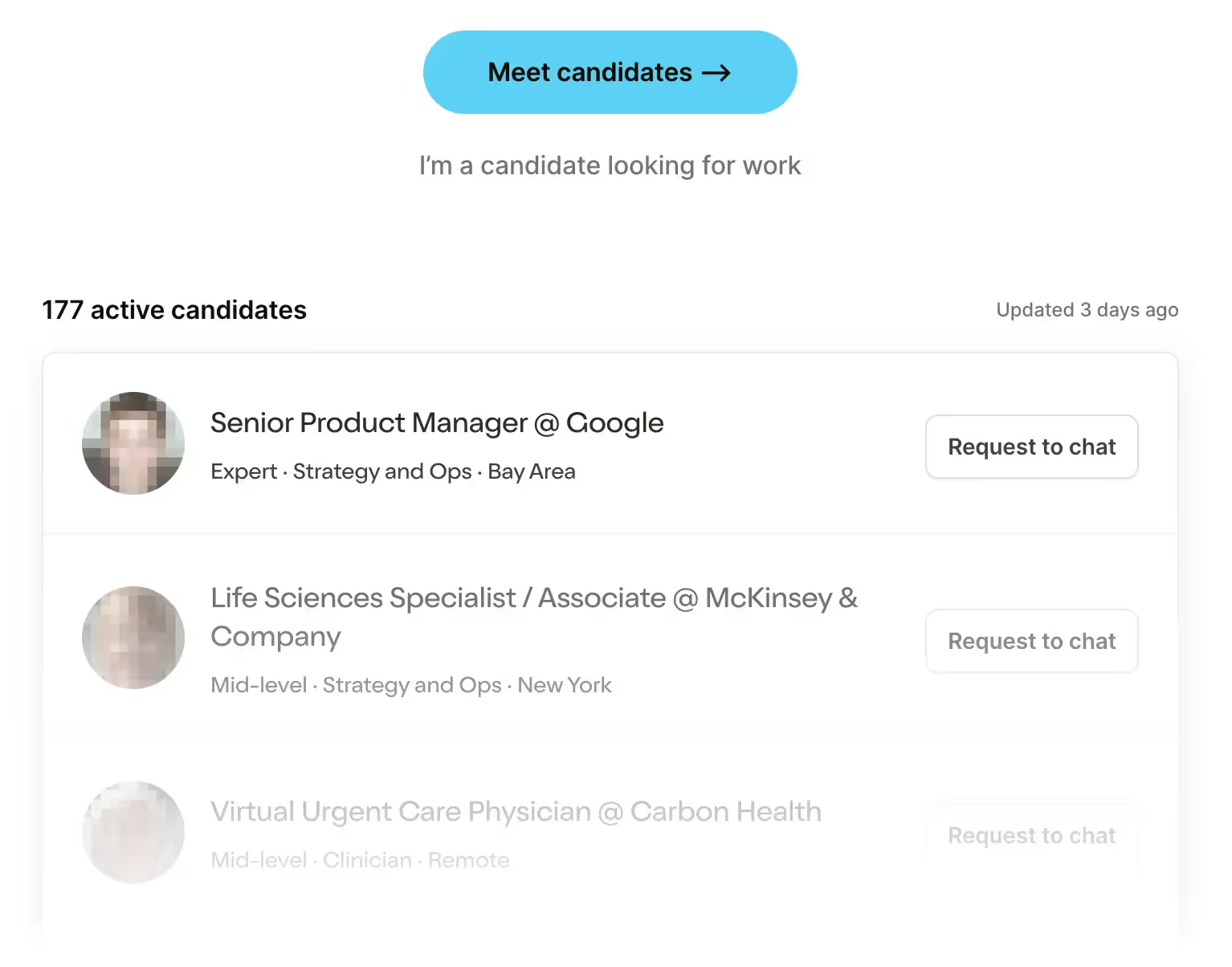
Featured Jobs
Finance Associate - Spark Advisors
- Spark Advisors helps seniors enroll in Medicare and understand their benefits by monitoring coverage, figuring out the right benefits, and deal with insurance issues. They're hiring a finance associate.
- firsthand is building technology and services to dramatically change the lives of those with serious mental illness who have fallen through the gaps in the safety net. They are hiring a data engineer to build first of its kind infrastructure to empower their peer-led care team.
- J2 Health brings together best in class data and purpose built software to enable healthcare organizations to optimize provider network performance. They're hiring a data scientist.
Looking for a job in health tech? Check out the other awesome healthcare jobs on the job board + give your preferences to get alerted to new postings.
Ayeeeee I
I’ve been having some good conversations about AI with different people. Most of the conversations have been around when we think people will try to have sex with the AI, and if our species will continue after that.
But occasionally there’s some healthcare AI conversations. So I wanted to get some thoughts down that are a little disparate. Here are a few “vignettes” of thoughts (it’s in quotes cause idk if I’m using vignette correctly).
- OpenAI and how AI healthcare companies can build defensibility
- How AI will create new types of data and what they might be
- Why AI in healthcare probably won’t reduce costs + an example
Open AI and the defensibility of AI companies
OpenAI had their recent demo day where they unveiled GPT-4o, a name that could easily be mistaken for a cell therapy in the pipeline. The whole demo reminds me of old Apple days at WWDC where every startup founder becomes temporarily religious as they wait to see if they release a new feature that would obliterate their startup.
You can see clips of the OpenAI demos here. They show a bunch of different examples including translation, two AIs talking to each other, being able to examine the world around them through video, and for some reason they “yassssss”-ified the personality of the AI.

There are 3 big things I took away from this:
Low latency. The AI responds near instantaneously to these prompts and the conversations are a hair shy of organic. The only part missing would be the AI making a joke while the other person is talking so they miss the punchline but laugh anyway out of politeness. This unlocks a whole new set of use cases for synchronous communication like automated phone calls, live assistance and interpretation, being able to interrupt the bot because you lack the self-awareness to know when you’re dominating the conversation, etc.
It’s 2x faster and half the price. The price is nearly low enough that it’s going to be cheaper than outsourced overseas talent, which can change the dynamics of a lot of businesses that depend on outsourced talent. I hope this isn’t like an Uber bait and switch on pricing, because I still yearn for those early days of $3 crosstown rides.
It’s inherently multi-modal. It’s interesting to see how the model has changed to be able to handle the low latency. And the answer is that it used to stitch a bunch of models together which caused a lot of lossiness and requires processing the prompt at each step which takes a few seconds. Instead, GPT-4o’s a totally new model with one neural network.
"Prior to GPT-4o, you could use Voice Mode to talk to ChatGPT with latencies of 2.8 seconds (GPT-3.5) and 5.4 seconds (GPT-4) on average. To achieve this, Voice Mode is a pipeline of three separate models: one simple model transcribes audio to text, GPT-3.5 or GPT-4 takes in text and outputs text, and a third simple model converts that text back to audio. This process means that the main source of intelligence, GPT-4, loses a lot of information—it can’t directly observe tone, multiple speakers, or background noises, and it can’t output laughter, singing, or express emotion.
With GPT-4o, we trained a single new model end-to-end across text, vision, and audio, meaning that all inputs and outputs are processed by the same neural network. Because GPT-4o is our first model combining all of these modalities, we are still just scratching the surface of exploring what the model can do and its limitations." - OpenAI
You know a company is doing bonkers stuff when this was a footnote of the presentation. The fact that this is a single model that can take inputs from all the different “senses” feels underrated. Once it can smell, humanity is doomed.
–
All of this does make you wonder what this means for healthcare. Does it make sense to build specialized healthcare models when these general AI models are getting so good? There are also increasingly more papers like this one that are examining multimodal models in healthcare as well. But does it need to be a healthcare specific multimodal model?
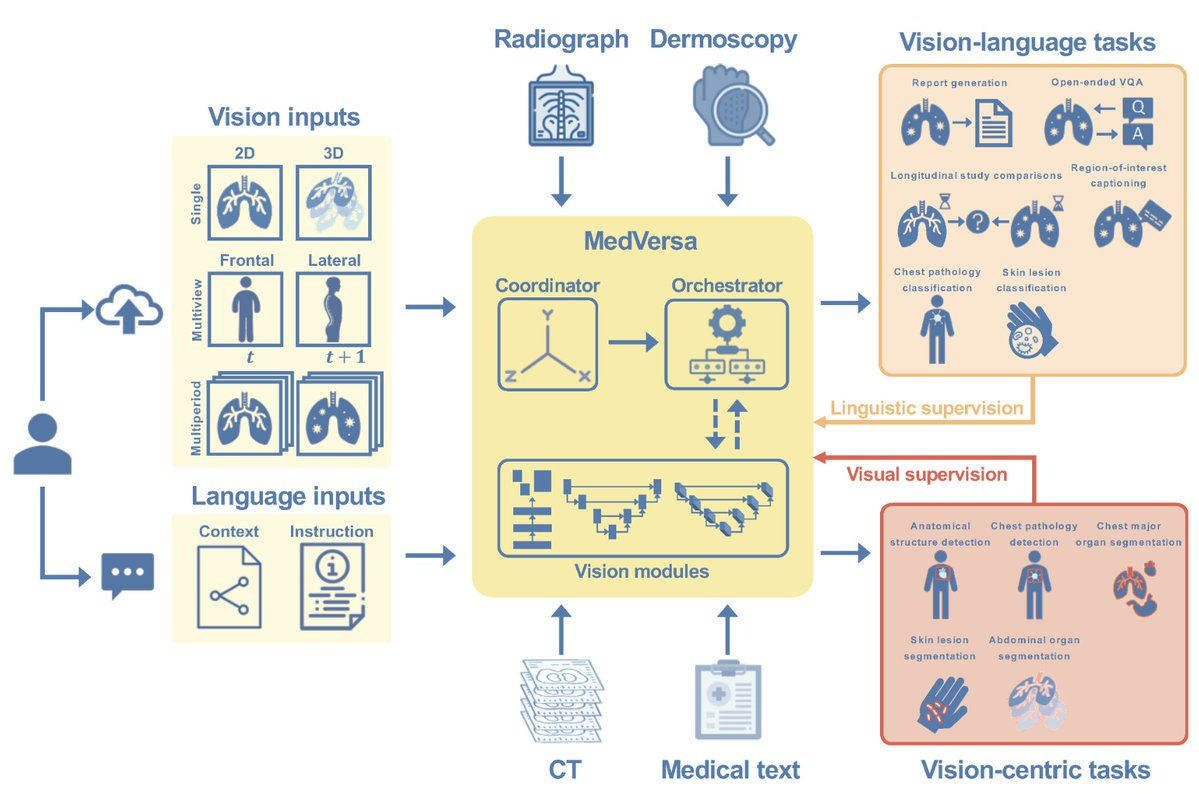
People I talk to that have deployed these models believe the gap between generalist and healthcare specific models only becomes evident when you deploy it in a real-world setting vs. comparing it to artificial benchmarks we see in demos and papers. The employer benefits market knows all about that! *sips tea*
So what does defensibility look like for AI healthcare companies? A few potential thoughts.
- Services companies. I’m increasingly convinced that value is going to accrue to companies that deliver services. This is partially because having a human in the loop can alleviate concerns with the AI making things up and all of the edge cases that tend to come up. But also because healthcare companies have more budget to replace headcount than they have budget to “invest in technology”.some text
- One version of this is micro private equity that acquires companies and then injects AI into their operations. Traditional success in acquiring healthcare businesses has been around negotiating better rates, controlling referrals, etc. But with AI maybe you can reduce the operating expense, improve margins, and just hold the business while it generates cash. This would probably work particularly well in areas where you need several full time staff to do administrative work like clinical trial sites, durable medical equipment suppliers, legacy third-party administrators, etc. Less so in places that have strict staffing ratio requirements.
- Another version of services is outsourced departments that’re powered by AI. For example, hospitals are now trying to completely outsource their billing departments (Allina outsourced it to Optum for example). A company can come in and be willing to take over all the headcount/back-office services themselves and do it for much less thanks to AI. For example, instead of 10 medical billers the hospital hires as full time employees, a company uses 1 medical biller + AI and charges 50% of the cost. Most of these just seem to be speeding up a bad process, but can still be good businesses.

- A third version is unbundling a package of services and completely automating part of it. A made up example, legal firms where you can choose between a lawyer reading a contract for $X or an AI they trained reading a contract for 20% of the cost. Or InPharmD, which is essentially a clinical pharmacist + AI which they use to create different products (ask a complex question about drugs, or for a hospital committee to generate reports on what drugs they should keep on hand).
- Local models. By “local” models I mean two things. some text
- Integrating a bunch of different local data sources at a system to help make decisions. Here’s an example of Viz.ai’s Hypertrophic Cardiomyopathy detection system. You can see in the workflow that it’s a combination of reading data, checking it against other types of patient data at the hospital, and then making workflow changes (e.g. scheduling). This can only happen with deep integrations at a per system level.
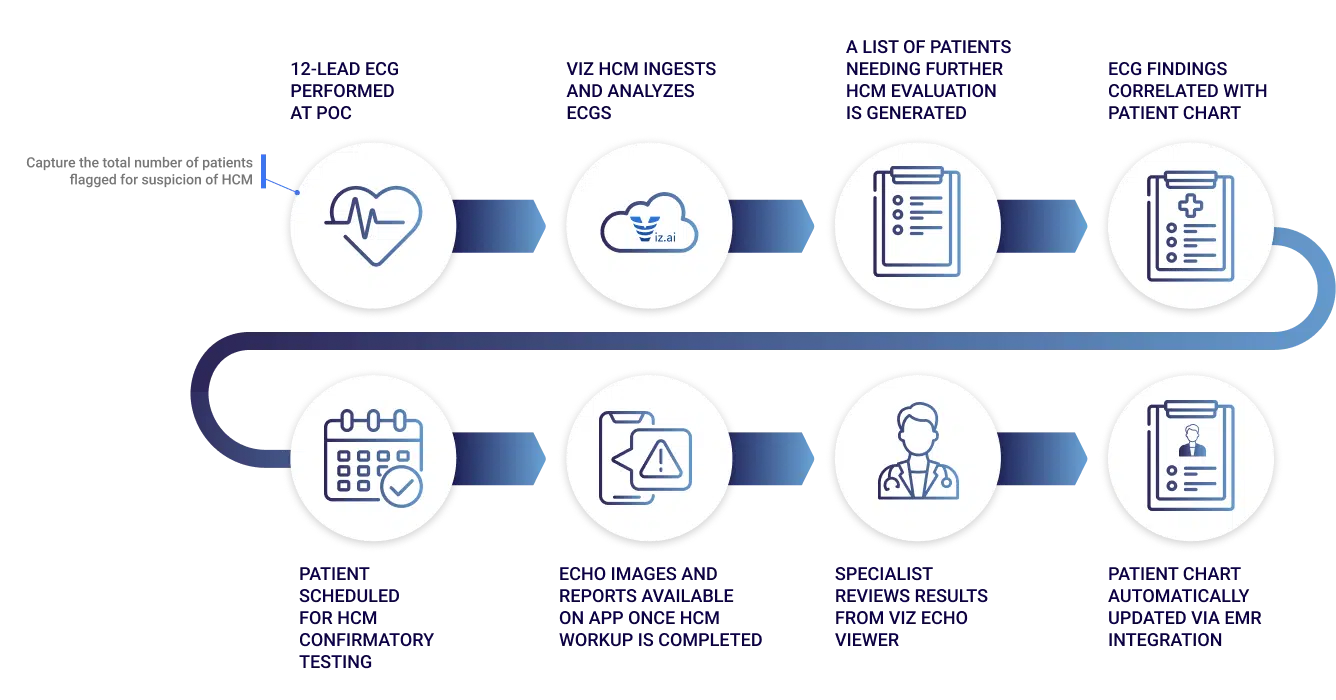
- Running local cloud hosted versions of models that then make easier to preserve privacy and mapped to your exact internal workflows. Here’s a paper where they built an EHR copilot agent that lives in the same hosting environment and within the same firewall as your EHR. The pros here seem to be that it’s privacy preserving and able to work without sending data beyond the network of a given hospital IT system. The cons are that it’s…really just okay accuracy-wise (this one only got 74% of tasks completed on the test they created) and it’s harder to audit/push updates to. My guess is that you’ll see some companies pop up that help set up local models, tune them to your setup, and then perform yearly tunings and audits. Then they’ll say “buy local”, with a smirk on their face.

- Hardware?- I know standalone AI hardware companies have basically been ridiculed to death. But I do wonder if there’s healthcare-specific AI hardware to be built. The issue with phones is that they aren’t always on, there’s a high friction of taking video or recording which AI can now interpret, they need to be physically taken out of your pocket to use, and they can be distracting since you see the rest of your life happening when you open your phone. But maybe purpose-built hardware can buit that is useful for surgeons in the operating room who can’t use their hands, or a more modern version of the in-hospital panic button, or even glasses powered by BeMyEyes that describe to visually impaired people what’s happening around them, etc.
—
Hackathon!
A quick interruption - hackathon planning is going great thanks for asking :). We have 60+ people hacking, 150+ people at demo day, a sick space, and some very good swag in the works. It’s in SF 6/22-6/23, as a reminder and you can see more about it here.
We have 2 sponsorship slots left. We already got ballers like Canvas, Abridge, etc. on board. If any of the following sound like you, email danielle@outofpocket.health for details.
- You’re a tool that engineers and data people building in healthcare should use
- You want to get in front of lots of people interested in starting a company or AI + healthcare
- You want to be an actual participant in the event, not a sponsor that sits on the side and hopes people see your logo.

Back to the post, but see how I kept it on topic???? It’s so native to the medium, which could be you…future hackathon sponsor.
—
AI increases data production
As generative AI tooling gets new senses and abilities, it also means people start pointing computers in different directions they previously didn’t. This means that a massive amount of new data is going to be captured/created because they know the computer can interpret it.
We’ll see:
- An increasing the volume of existing datasets
- Getting data out of separate systems and into a centralized place
- The creation of entirely new types of data that didn’t really exist before.
Some examples:
Voice-to-text - Today, a doctor sees you and writes notes from the encounter into your record. This is very lossy because the doctor only writes down what they think is relevant and important (especially for billing). AI scribing companies now have a record of the raw audio data which can be analyzed.
Robocalling for data - The ability to automate phone calls is basically an API to get information about the real world without needing to integrate with anything. It’s kinda like faxing except both parties don’t need fax machines or people in a retirement home that know how to use them.
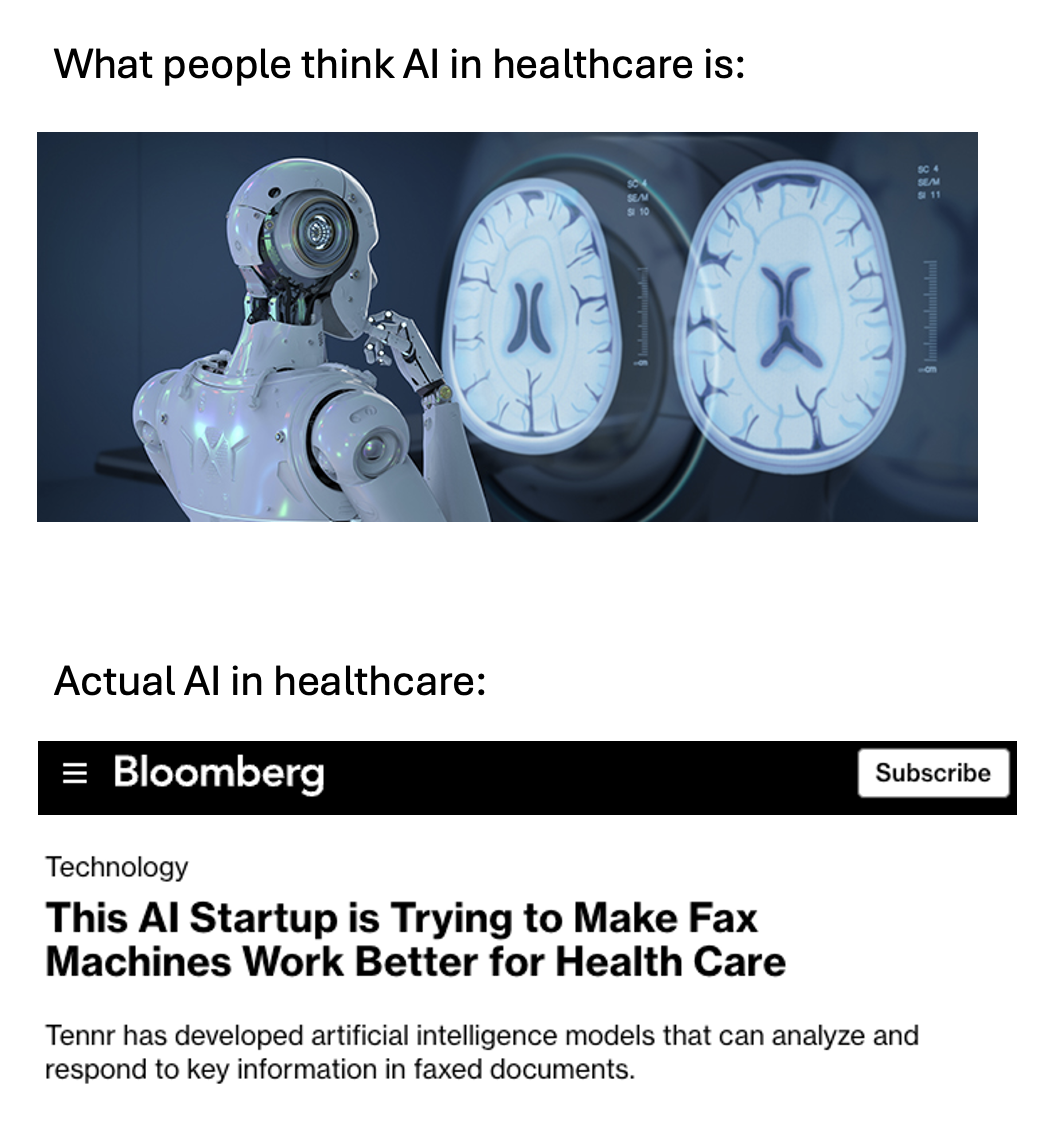
Robocalling allows for much more flexibility and chaining of requests to get specific information. You can call a doctor’s office to find out their next appointments and hack together a universal calendar patients can see. You can call a local pharmacy to see if something is in stock. Or you can ask your insurance company if a specific procedure is covered under a plan. New businesses are going to be built that just robocall places to get information and build databases of the answers.
Video data - Now that AI has “machine eyes” essentially, a lot of people are going to start recording more things and asking the AI to interpret it. We’re already seeing AI analysis of existing video like endoscopies (e.g. Virgo), children’s behavior (e.g. Cognoa), and physical therapy exercises (e.g. Kaia). But now I think people will start recording even more videos and ask AI about it later. A camera that watches the patient during a visit and lets the doctor know if they missed something visually. People recording their parents at home to watch for falls but also ask an AI if there’s some change in their gait. Even things like recording a workout and seeing how your body is changing as you do it.
Sound data - There’s a lot of data that we listen for in a healthcare visit. Heart and lung sounds are captured in a stethoscope but rarely recorded (Eko has a digital stethoscope). What about the sounds our joints make? Or voice tremors when we’re talking? Or sentiment analysis of patients when you call them? Maybe people start recording these sounds themselves to see if the AI can make sense of it without needing to go to a doctor for the interpretation.
–
It’s cool to think about what other activities we can turn into actionable data in a healthcare setting nowadays. The open question though is figuring out if the business model is selling data, creating better models, or something else.
Does AI in healthcare reduce cost?
There’s a good discussion here about why AI hasn’t and probably won’t structurally lower the cost of healthcare. A lot of people had good takes around needing to totally reimagine care workflows, skepticism from decision makers in the industry, etc.
It’s a good question - personally I think it’s three-fold.
- Healthcare markets are not competitive, so any AI that lowers operating costs does not reflect in lower prices. It just reflects in better margins for whoever uses it.
- Losing revenue in one service line means that costs will increase somewhere else to offset it.
- Healthcare AI only becomes used if it can induce more patient demand, bill more, or will allow you to lay people off/not hire more people for a role.
An interesting place to examine this dynamic is diagnostics. A handful of companies have been able to offer fully automated analysis of different types of scans without a doctor in the loop. There’s a great analysis here about how these companies are billing and being used.
Now before you continue reading, which do you think is more expensive: a scan where the software makes a diagnosis or a scan read by a doctor to make a diagnosis?
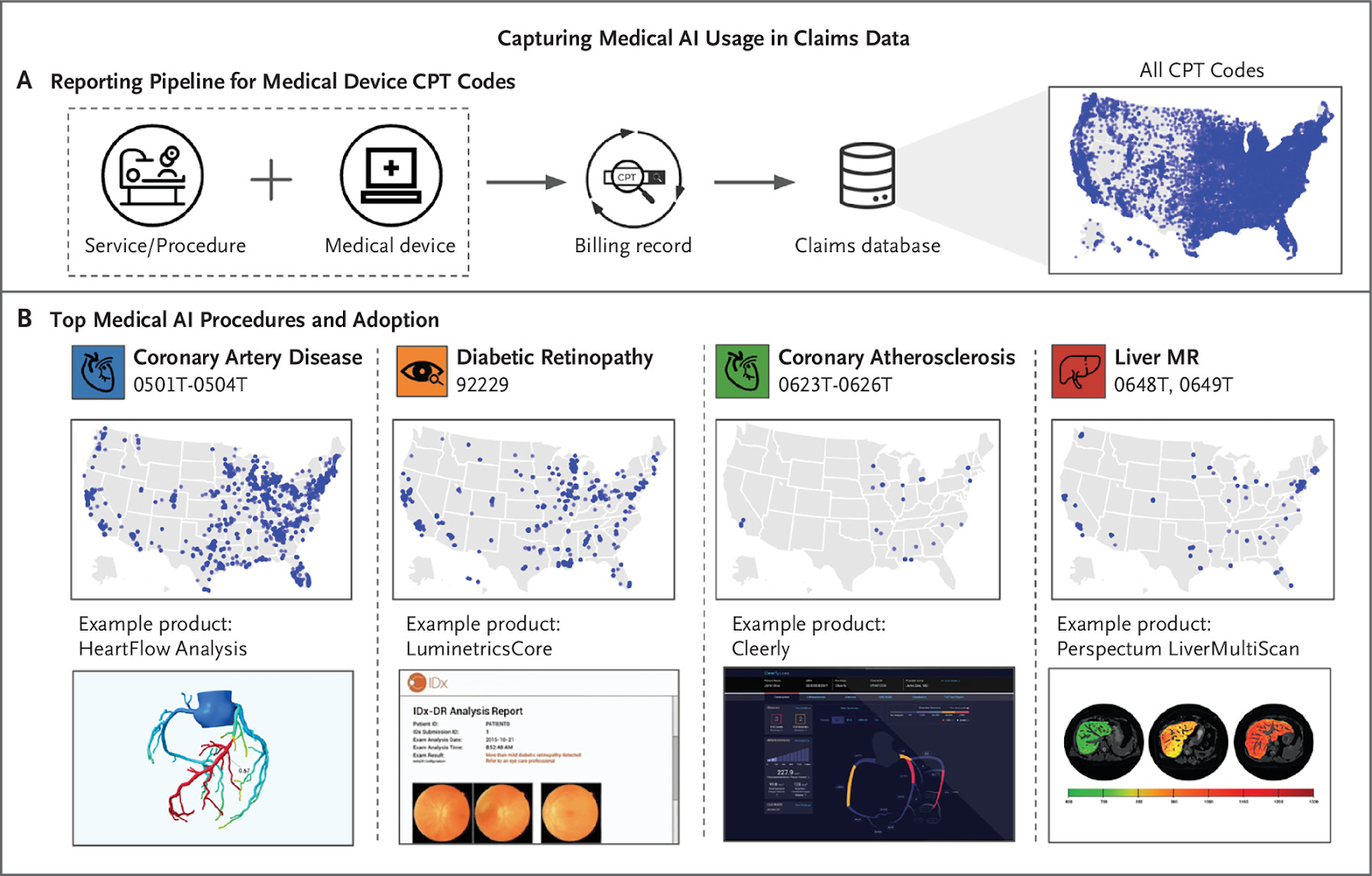
Personally my dumbass tHouGhT fRoM FiRsT prINcIpLeS and figured by removing the doctor from the equation and instead using software which costs ~$0 to do the analysis, this would mean that the reimbursment would be lower. But actually it’s the opposite - AI scans seem to be more expensive than their non-AI counterparts.
The quintessential example of this is screening for diabetic retinopathy. In 2021 they created a CPT code 92229 for an AI to do an automated analysis of your eyeballs. Medically it’s called your fundus, which is also what the screening companies said to the VCs.
Normally a doctor analyzes the image, but for the first time a practice could get reimbursed with an AI interpreting the image without a human in the loop. Except the AI interpretation is more expensive than the doctor interpretation.
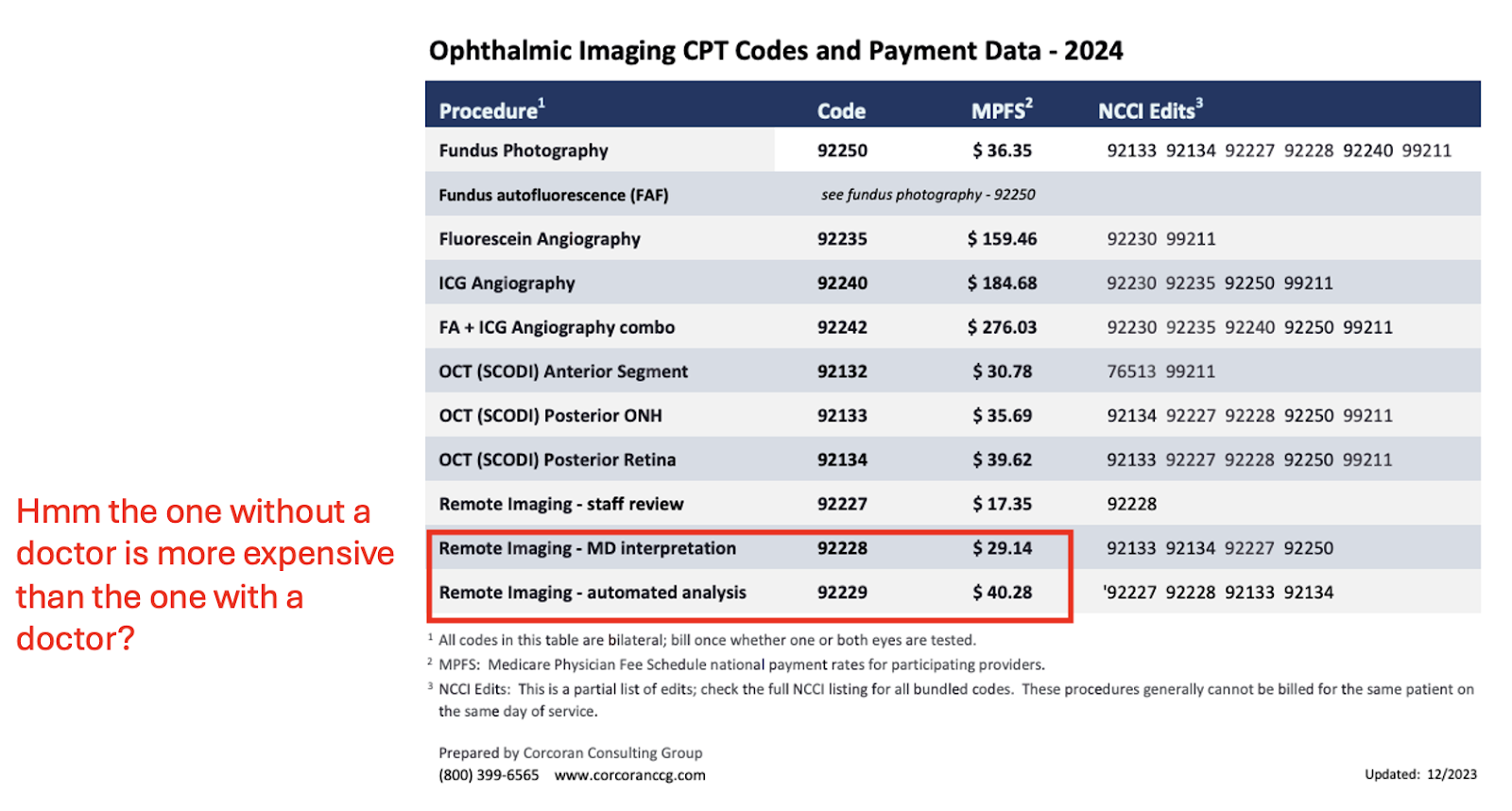
The rationale the AI companies make to insurance companies is “we should get paid more because the automated system will catch more patients earlier and save you costs down the road”. The surgery to fix this is $4-6K+, so catching patients early is important and many are undetected. This is especially true because an AI-only interpretation can be done in a primary care setting, which will catch way more patients vs. needing them to go to an eye doctor specifically. But the cost these primary care or retail settings have to pay for the machines alone to do this analysis seems to be between $9K and $16K+ depending on the image quality, so they need to get enough reimbursement to make that investment worth it.
I do understand this argument. It’s probably a good thing that more patients get access, more dollars go to primary care through this, and more diabetic retinopathy gets caught. In fact, AI might reduce cost in the longer run if it can reduce the number of those surgeries.
This is emblematic of why AI in healthcare doesn’t reduce cost.
- The doctors and hospitals are largely in control of which CPT codes get approved and therefore what gets paid for. You think they’re going to approve codes for any AI that’s potentially a threat?
- The ones that do get approved need to be reimbursed enough money for them to actually get adoption.
- Payers decide if the AI is valuable enough that they should pay for it. If it does save money it likely won’t flow to you as the patient because you’re usually not their customer, employers are.
This dynamic is also not limited to diabetic retinopathy, but many AI screening tools:
“For example, AI interpretation of breast ultrasound (CPT codes 0689T–0690T) has a median negotiated reimbursement rate of $371.55, which is comparable to the national average cost of a traditional (non-AI) breast ultrasound of $360.74 However, AI analysis of cardiac CT for atherosclerosis has a median negotiated rate of $692.91, which is higher than the average cost range of a cardiac CT of $100 to $400.75” - NEJM
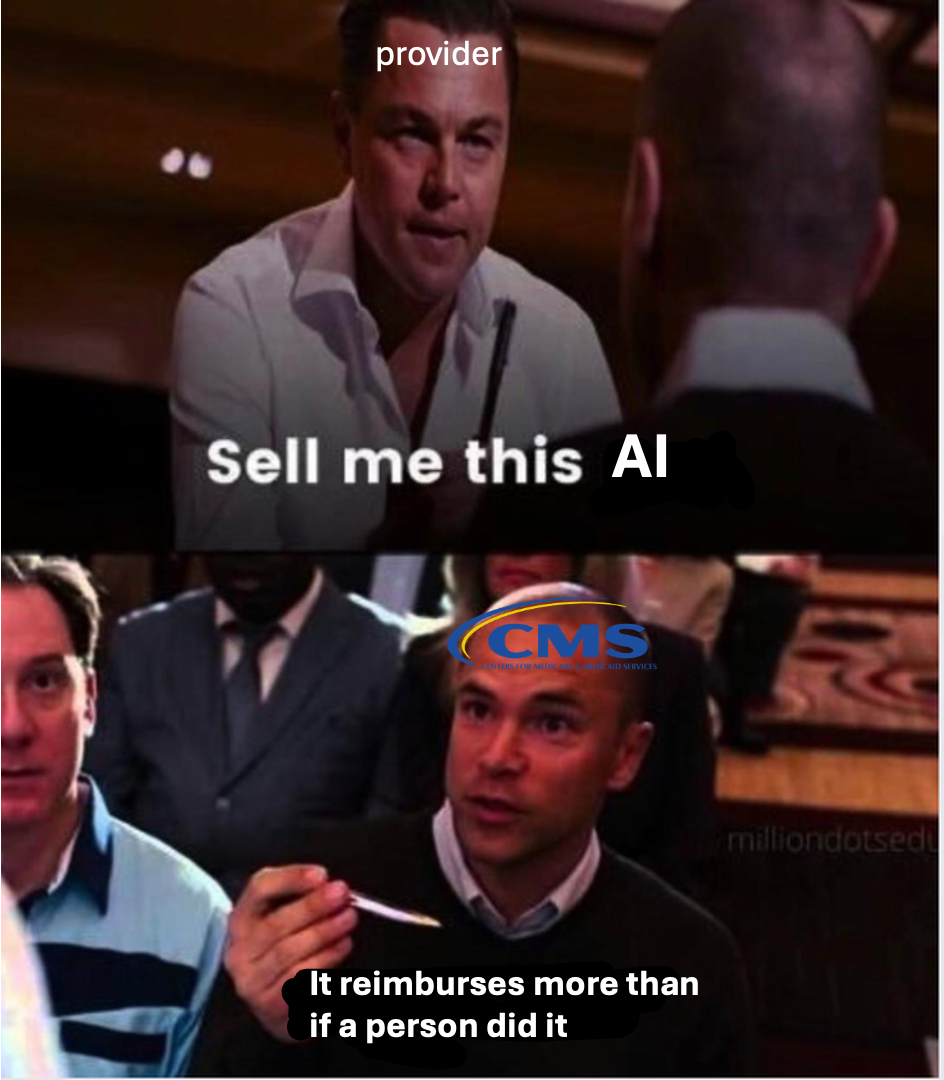
A parallel universe is consumers paying out-of-pocket themselves and bypassing this entire process. For example, Radnet has an AI called Saige-Dx that helps radiologists detect more subtle lesions but patients have to pay $40 out-of-pocket for it. They were even piloting these at Walmart (whoops!).
It’ll be interesting to see if the cost of this service goes down over time as it competes for more customers. But will patients that need this can choose to pay out-of-pocket for it vs. other expenses in their life? This presents a different set of pros/cons.
In general I don’t think AI will end up reducing costs to patients in healthcare. But as we’ve seen with the diabetic retinopathy screens, it might make care more accessible for more people and improve outcomes. That’s still good right?
Parting thoughts
Yeah…my kid is going to have an AI boyfriend/girlfriend aren’t they.
Thinkboi out,
Nikhil aka. “just a meatsack of training data”
P.S. Knowledgefest applications are due on Monday, don’t forget.
Twitter: @nikillinit
IG: @outofpockethealth
Other posts: outofpocket.health/posts
Thanks to Phil Ballentine and Morgan Cheatham for reading drafts of this
--
{{sub-form}}
If you’re enjoying the newsletter, do me a solid and shoot this over to a friend or healthcare slack channel and tell them to sign up. The line between unemployment and founder of a startup is traction and whether your parents believe you have a job.
Interlude - Courses!!!
See All Courses →We have many courses currently enrolling. As always, hit us up for group deals or custom stuff or just to talk cause we’re all lonely on this big blue planet.