The Reality of Real-World Evidence
Get Out-Of-Pocket in your email
Looking to hire the best talent in healthcare? Check out the OOP Talent Collective - where vetted candidates are looking for their next gig. Learn more here or check it out yourself.

Claims Data 101 Course
.gif)
Featured Jobs
Finance Associate - Spark Advisors
- Spark Advisors helps seniors enroll in Medicare and understand their benefits by monitoring coverage, figuring out the right benefits, and deal with insurance issues. They're hiring a finance associate.
- firsthand is building technology and services to dramatically change the lives of those with serious mental illness who have fallen through the gaps in the safety net. They are hiring a data engineer to build first of its kind infrastructure to empower their peer-led care team.
- J2 Health brings together best in class data and purpose built software to enable healthcare organizations to optimize provider network performance. They're hiring a data scientist.
Looking for a job in health tech? Check out the other awesome healthcare jobs on the job board + give your preferences to get alerted to new postings.
If you’re enjoying the newsletter, do me a solid and shoot this over to a friend or healthcare slack channel and tell them to sign up. The line between unemployment and founder of a startup is traction and whether your parents believe you have a job.
Spurred by a spat between Keith Rabois and Zach Weinberg, last week I talked about randomized controlled trials and their shortcomings. Congrats if you read the whole thing, I didn’t even read the whole thing.
It was meant to give a basic understanding of why randomized controlled trials are important. Now I can get to the core of their actual debate: when is observational data (aka. real-world data) useful?
*As a quick terminology point, real-world data is the data itself while real-world evidence is the usage of that data to actually prove something. I’m going to use real-world evidence throughout the entire post to make it easier.
**This post is going to be focused on the use of real-world evidence for biotech and pharmaceuticals. Medical devices, diagnostics, and screening tools have some slightly different nuances.
Observe
Should we be running a randomized controlled trial on every single thing we do?
Nah. We know for a fact that some things have direct causation, we don’t need a trial to prove it. We know if it’s good or not based on observation. Actually this is how medicine first started - people would notice things that worked, try them out, and then those recommendations would spread.

But at what point do you KNOW something is good or bad? When two things seem related, we all know to appropriately and smugly say “correlation does not imply causation” while basking in our clear intellectual superiority. But there needs to be a point at which we DO infer causality, right?
Keith is right in that there was no randomized controlled trial done to link smoking and lung cancer. After a few papers showing the harmful effects of tobacco in animals, a 1954 paper by Sir Richard Doll and Sir Bradford Hill showed such a strong correlation between smoking and lung cancer in humans via observational data that the scientific community agreed that causality could be inferred. Several other epidemiology studies came out around the same time with similar results.
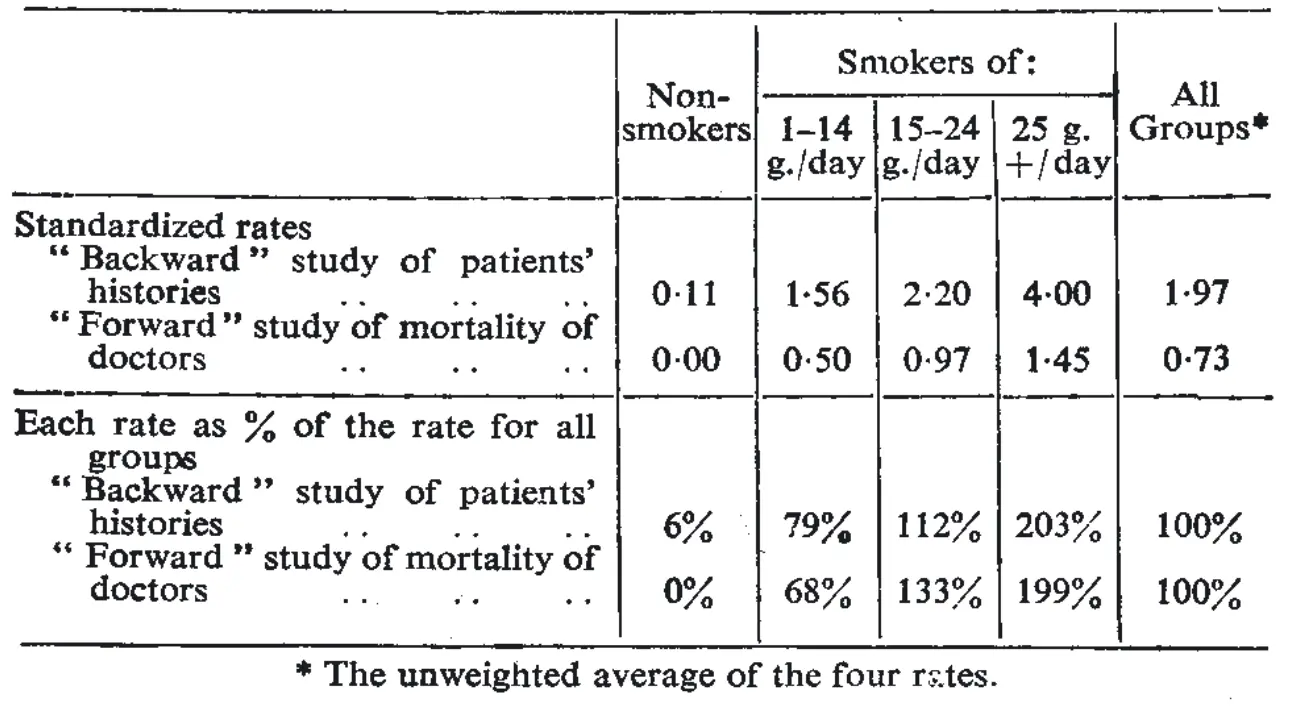
The author of the paper, Sir Bradford Hill, established 9 principles to figure out when correlation should imply causation if you’re looking at the relationship between some intervention/environmental factor and a disease:
Strength Of Association - Pretty self-explanatory. Is the strength of association between the two strong or weak?
Consistency - Is the association between the two factors found consistently?
Specificity - Is there a 1:1 relationship between exposure to X that causes Y disease. Today we know that exposure to different factors can cause a multitude of different diseases, but at the time it was believed the relationship was more specific.
Temporality - Did the exposure happen before the disease manifested? (bonus points if the disease manifests in a consistent amount of time post-exposure)
Biological Gradient - Does more exposure yield more severe versions of the disease?
Plausibility - Can the relationship between exposure and disease be explained? Is the mechanism actually understood? Even Sir Bradford Hill said this probably wouldn’t be possible in most cases.
Coherence - Does that mechanism/reason for association defy what we know previously about how this disease usually manifests?
Experiment - Do we have any other experiments we can point to (e.g. animal models).
Analogy - Are there other exposure/disease relationships we can point to that are similar?
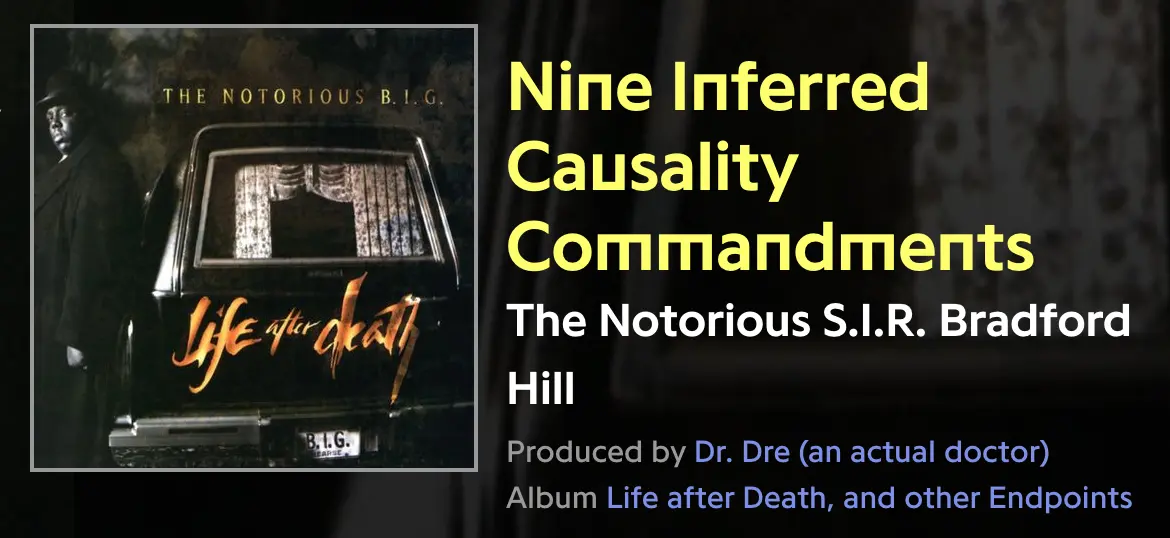
These have been debated over time, but a useful framework. If we do not have enough evidence via these criteria, a randomized controlled trial would help establish a more clear relationship.
Also the kicker? Sir Bradford Hill ALSO pioneered the randomized clinical trial! Find someone that looks at you the way he looked at experiment design.
Establishing the link between smoking and cancer used observational data instead of a randomized controlled trial to make this conclusion. So while Keith is correct that a randomized controlled trial was never performed, that exact situation is actually why we had to set up frameworks to understand when observational data is actually causal. And it turns out the bar to make that claim is quite high.
The Cool Things You Can Do With Real-World Evidence
Today, observational data is more abundant than ever thanks to the (glacially slow) digitization of healthcare. Real world-evidence encompasses everything from electronic medical records, pharmacy data, insurance claims, data from your phone usage, and more. It’s really any dataset that can tell us about more about your health.
The FDA has been very on board with using real-world evidence. The 21st Century Cures Act of 2016 placed a strong emphasis on using data from actual hospitals and practices to complement existing clinical trials. Some cool ways it’s being used today:
Speeding up randomized controlled trials + monitoring long-term outcomes
A common trope I hear is “FDA bad, they make good drug take long time”. The reality is that the FDA is testing for two things when they look at a drug, safety and efficacy. For diseases where no good drugs exist, a new drug that shows efficacy (and a basic measure of safety) in a randomized controlled trial can actually get approved pretty quickly. If the patient is going to die anyway then efficacy matters more than safety.
However, if a drug IS fast tracked the FDA will ask for long-term tracking of patients to make sure there are no safety issues with that drug down the line. This is called “Post Marketing Data” or “Post Approval Data”, and real-world evidence is very important here.
By COMBINING a randomized controlled trial with real-world evidence, we can bring drugs to market faster.

The real problem is actually getting the drug companies to submit that post-marketing data on time.

Scale + Diversity Of People
A big benefit of real-world evidence is how much larger and more diverse the datasets are. Data can be can be pulled directly from existing sources vs. a clinical trial which has its own logistics and generates its own data.
With a larger dataset, we can hone in specific subgroups of patients that respond particularly well/poorly and see if there’s any reason why that might be the case.
We can also analyze groups that may not be well represented in the trial because of their rarity. A drug called Ibrance recently got approval from the FDA to expand from metastatic breast cancer in women to male patients by using real-world evidence instead of a traditional randomized controlled trial.
This was a great example of using real-world evidence because it was a rare population with potentially high variance in how they would respond to the drug.
FDA has previously extrapolated efficacy and safety by granting indications in breast cancer to male patients, even if no male breast cancer patients were enrolled in the supporting trial.
However, in certain cases when there is the potential of differential efficacy or safety results between men and women with breast cancer, such as when a drug is combined with endocrine therapy and results in or relies upon manipulation of the hormonal axis, further evidence may be necessary to support a labeling indication for male patients.
This need for additional evidence, combined with the rarity of male breast cancer, made this the right setting to use [Real World Evidence].
-FDA
Using Existing Drugs For New Diseases
In case you didn’t know, while the FDA approves a drug for a specific disease, doctors can actually prescribe drugs for whatever diseases they want. If a patient is out of options, a doctor might try some treatments based on some papers they’ve read. This kind of prescribing is called “off-label use”.
This is what’s happening with chloroquine and hydroxychloroquine. These are drugs approved for malaria and inflammatory disorders like rheumatoid arthritis but are being prescribed off-label to treat COVID-19.
Real-world evidence can reveal how drugs work well for other diseases by monitoring their off-label usage. Or if those drugs work surprisingly well in combination with other drugs/interventions. That kind of data is informative in designing future randomized controlled trials for that drug.
Look at how many diseases the medication Humira has been approved for over time. If it ain’t broke, try another autoimmune disease.
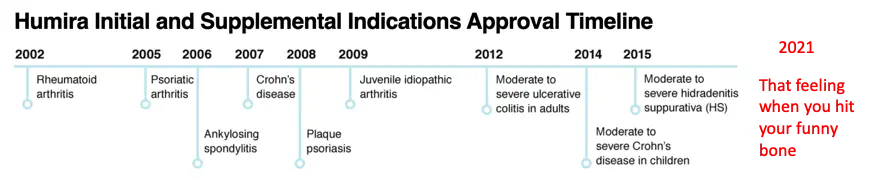
New Trial Designs
The emerging and interesting benefit for real-world evidence is different trial designs you can conduct. Some examples:
Single-arm trials and synthetic controls - If a disease is rare, it’s already going to be difficult to find patients for your trial. Using real-world evidence, you can actually compare your experimental treatment against people that are just getting their regular treatment, and act as if they’re a control arm.
In this case, you only need to recruit the arm that’s getting the treatment to the trial. This cuts the number of patients you need to recruit in half, and every patient recruited knows they’re going to get the new drug.
This is one of the areas Flatiron Health has done quite a bit of research in.
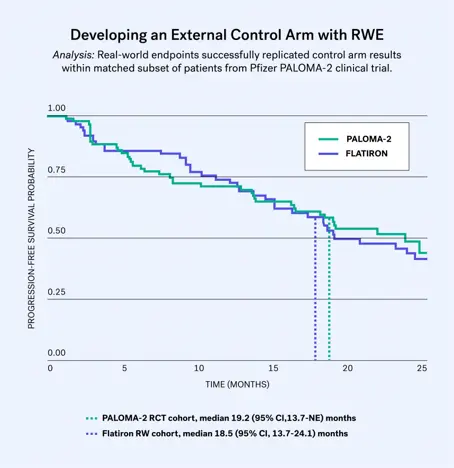
You can even take this one step further, and create “synthetic” control arms from patients in the past. Imagine you took all the data from control arms in previous clinical trials, or records of patients that had a rare diseases that you tracked for the entire course of their life. You could use that data as the comparison instead of waiting for patients today. This gets very dicey because how the disease is treated can change over time, or you could cherry pick historical patients, etc.
Replacing a control arm is doable if a disease tends to manifest and progress in a similar way each time. If someone has a deadly disease that tends to kills virtually everyone that has it after 2 years, we can pretty comfortably say the disease is what killed the patient. So if a drug causes patients to not die after 2 years, we can pretty comfortably say the drug had an effect. Because the attribution is very clear, you don’t need to recruit a control arm because we already understand when the drug is working. Plus it would be pretty unethical.
Pragmatic and hybrid trials - Pragmatic trials aim to figure out if there are practical improvements to be made when giving drug or intervention. The focus is more about making policy or changes in how the medicine is given rather than figuring out if a new medicine works.
For example, this study examined using a monthly injectable anti-schizophrenia medicine vs. a daily oral anti-psychotic in patients with criminal history. These real-world patients were randomized and monitored. They found the injectables were better because of better adherence for that specific group by using a real-world setting.
Hybrid trials are being increasingly explored, which combine regular randomized controlled trials with pragmatic trials. For example, a new drug might start with a small randomized controlled trial to test for efficacy in a disease to get approval and then rollover those two arms into the real-world to recruit many more people. Or a trial might be designed to test for one indication, and then after it proves it works in that disease immediately start recruiting real-world patients for effects in new diseases. This paper has a lot of cool potential examples.

Adaptive (field?) trials - So I saw this part of the exchange and was confused. You can use a large real-world evidence trial sometimes instead of a randomized controlled trial in some really specific cases, like the Ibrance example I gave before (which Zach’s company Flatiron Health actually powered lol).

But for new drugs I don’t think you can SUBSTITUTE a randomized controlled trial with a real-world evidence trial yet. However, there is one scenario where I know this happened. And it was during the Ebola outbreak, making it particularly relevant here.
The study design for the ebola vaccine Ervebo was interesting and super controversial. They designed a “ring vaccination trial”, where they recruited patients that were at high-risk of exposure based on the degrees of separation from the infected. Then, they put patients in one arm and gave them the vaccine immediately, while the other arm waited 21 days. They then compared those two arms against each other, there was no real placebo arm.

There’s a lot of controversy over whether the vaccine actually worked. It’s also possible that it did work, but with really low efficacy. Honestly, impossible to tell without a true control arm.
This ring design is a type of adaptive trial, where you learn something during a trial, and then change an aspect of the trial in the middle. However these rules have to be pre-specified, you can’t just make that shit up on the fly. In pandemic scenarios like this, we can potentially use real-world evidence to create some of these unique, adaptive trial designs.
The Obstacles Of Real-World Evidence
As I’ve painfully learned through the years, nothing in this world is 100% good except Breaking Bad. Here are the problems with real-world evidence.
The patients aren’t random
I already went over this ad-nauseam in the last newsletter. Patients in the real-world aren’t randomized, so you’ll deal with selection bias issues.
The data inputs are suspect
When you start reading about healthcare you’ll be flooded with acronyms but there’s only one that matters: GIGO (Garbage In, Garbage Out).
Data coming from the real-world has an entire host of problems.
- There’s a lot of variance in how doctors practice, so how they treat diseases might be very different.
- If you’re using insurance claims or billing data, there are lots of issues with how doctors code. Remember, this data comes from doctors telling insurance companies how much they should get paid…you think that’s going to be flawless? Charging up, not coding at all because they aren’t going to get reimbursed, etc. are all huge issues here.
- Doctor’s notes in the medical record are arguably the most valuable piece of data, but have you ever read a doctor’s syntax? There needs to be a Rosetta stone course to decipher it.
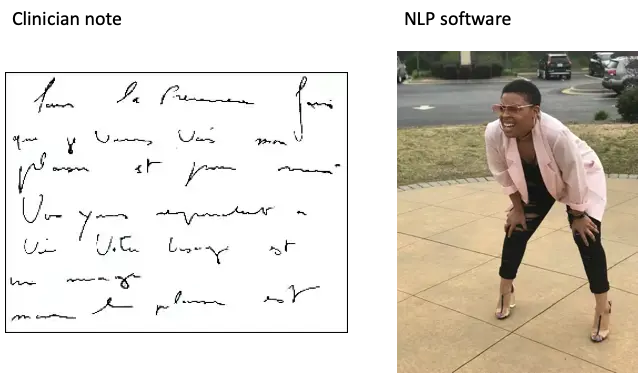
Data harmonization
Sure there’s all this data but it’s f***ing impossible to actually get it all to sync together. How do you know it’s the same patient across the data sources? What data standard should all of these sources map to? Why the hell do I still have to get the images on a CD-ROM?
This paper came out a while ago that showed some of the linkage process between Flatiron Health and Foundation Medicine. These are two companies are owned by the same parent (Roche) - look how many steps are required to get these two datasets to talk to each other. Now imagine it’s 10 different data types, they’re each independent, and research isn’t their core business.

Data is missing
To do a true, objective, and thorough test of a new drug there’s a lot of information you have to capture about patients at every single visit at regular intervals. Everything from getting labs done, doing a set of procedures (e.g. an endoscopy), etc.
The reality is that in the real-world, doctors are not capturing this kind of data as frequently. Many times there are financial blockers like insurance coverage, the physician simply doesn’t feel those additional tests actually add anything, etc. The absence of that data means you might be missing key information that’s important to understanding how a drug works.
A study looked at how many clinical trials could be theoretically replicated using only real-world evidence. The answer? 15%. Most of the data you would need to run the trial just wasn’t available in the real-world.
Honestly 15% is higher than I expected. But it’s indicative of how research and everyday care have been historically kept separate, and we’d have to blur the lines between the two if we want to get more value out of real-world evidence.
Conclusion - Who Was Right?
I am.
Just kidding.
There is no 100% correct answer here, basically every hyperbolic statement was wrong on all sides. Randomized controlled trials and real-world evidence are fantastic complements to fill each others gaps. One gets data from small groups with theoretically less statistical bias and tests very specifically for safety and efficacy, the other gets noisier data from large groups to teach us new things about drugs when they’re used at scale in practical settings and informs future trials. One isn’t inherently better than the other, it just depends on what questions you’re trying to answer.
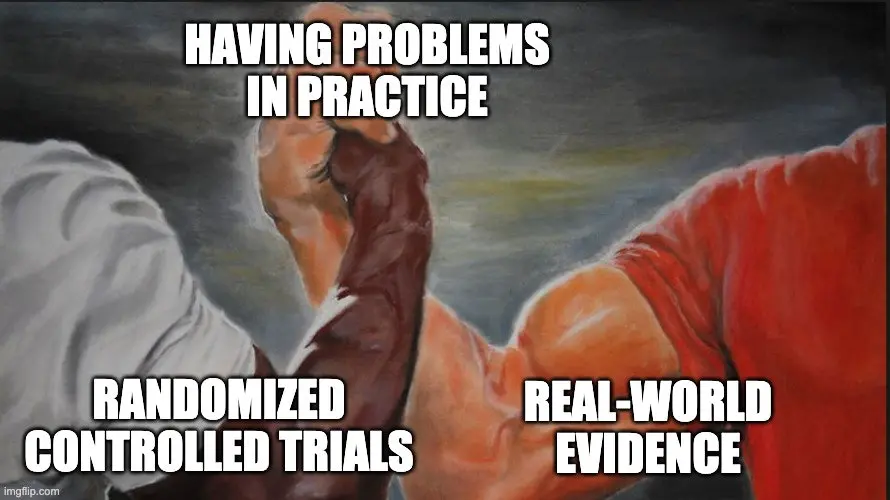
Some things that are worth keeping in mind during this debate.
- Urgency - It’s easy for us to conceptualize the issue with letting unsafe drugs into the market because we can see the people directly hurt by it and can punish a specific company that endangers people. But there are lots of people who are being hurt because of how slow it is to bring a drug to market, which is harder to conceptualize and punishment isn’t really possible. How can we understand when bucket 2 is worse than bucket 1?
- Prevention vs. Intervention - There’s a very big difference between taking a drug in anticipation of a disease and taking a drug when you have it. The scale of people taking something preventively is massive, so our bar for safety has to be much higher. What if taking a drug for prevention actually puts you more at-risk?
For people taking a drug when they have the disease, the risk of the disease itself has to be weighed against the risk of the treatment. Hydroxychloroquine + azithromycin has the potential to throw your heart rhythm out of wack, so if you have underlying heart issues that could be a problem. This is particularly problematic considering COVID-19 has a higher mortality rate for patients with underlying heart issues. - Opportunity Cost - Testing a treatment for a patient means we’re preventing them from being on another potentially better treatment. The reality is you can’t try every therapy in every patient, so if we’re going to test we should have a good understanding why.
I know this might be kind of crazy to say but…I have no idea if hydroxychloroquine is a good therapy because I’m not an expert. As more studies come out we can answer more of Sir Bradford Hill’s criteria. But unless we perform a real randomized controlled trial we won’t know for sure, and we should view the shortcomings of each study that comes out to understand what questions are still open so that future trials may provide answers.
As a society we need to make a decision on how much we care about statistical rigor vs. speed to address the current situation, and it’s clear that each country is making their own decisions around this.
While my answer about who won the debate might be unsatisfying, I’m glad it provided an opportunity to talk about the different ways to test drugs. If the benefit of Zach and Keith’s shit-flinging was more people learning about how trials work, I think that’s a positive.
Maybe the real evidence was the friends that blocked each other along the way.
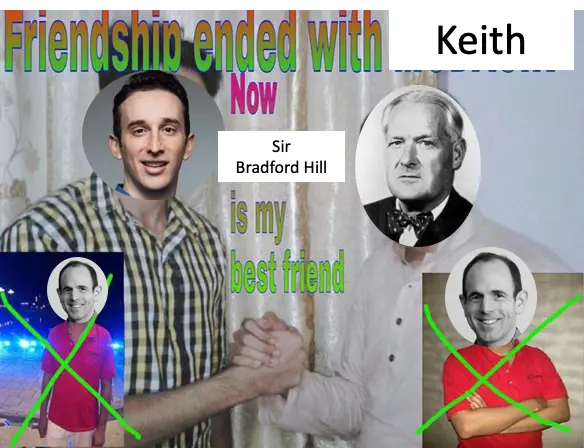
Thinkboi out,
Nikhil aka. The World’s Realest Evidence