Random Uncontrolled Trials/Tweets
Get Out-Of-Pocket in your email
Looking to hire the best talent in healthcare? Check out the OOP Talent Collective - where vetted candidates are looking for their next gig. Learn more here or check it out yourself.
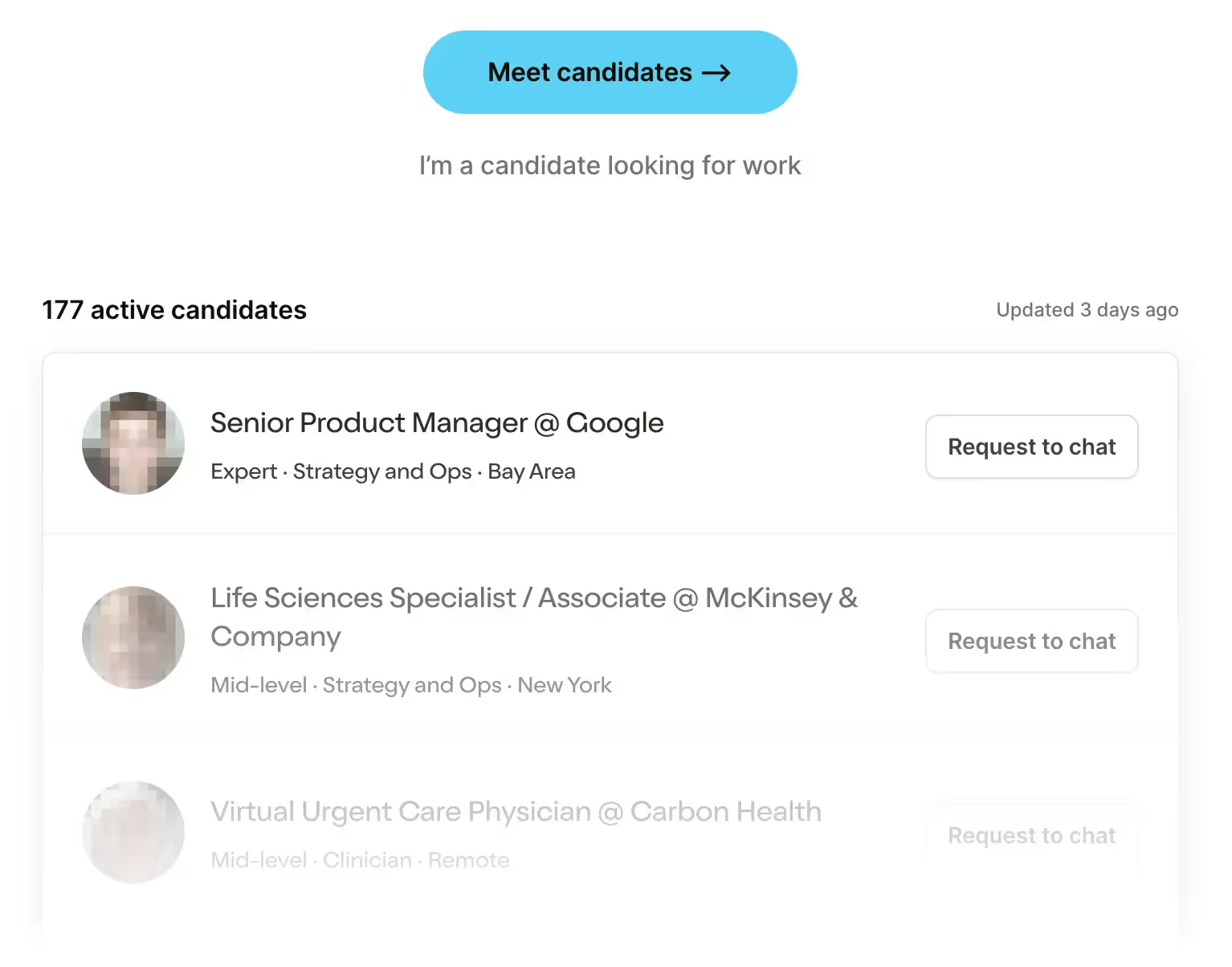
Featured Jobs
Finance Associate - Spark Advisors
- Spark Advisors helps seniors enroll in Medicare and understand their benefits by monitoring coverage, figuring out the right benefits, and deal with insurance issues. They're hiring a finance associate.
- firsthand is building technology and services to dramatically change the lives of those with serious mental illness who have fallen through the gaps in the safety net. They are hiring a data engineer to build first of its kind infrastructure to empower their peer-led care team.
- J2 Health brings together best in class data and purpose built software to enable healthcare organizations to optimize provider network performance. They're hiring a data scientist.
Looking for a job in health tech? Check out the other awesome healthcare jobs on the job board + give your preferences to get alerted to new postings.
If you’re enjoying the newsletter, do me a solid and shoot this over to a friend or healthcare slack channel and tell them to sign up. The line between unemployment and founder of a startup is traction and whether your parents believe you have a job.
*record scratch*
*freeze frame*
Yup, that’s me. You’re probably wondering how I ended up in this situation.
Getting caught in the middle of two well-known tech people arguing about the merit of randomized controlled trials because one blocked the other.

Before this argument rapidly devolved into mud slinging, it started as a pretty important topic:
- Why are randomized control trials important?
- What are the shortcomings of randomized trials?
- Are there times we should actually skip them or alternative trial designs would make more sense?
As with any Twitter argument, this very rapidly degraded and instead of anyone actually addressing the points it just became a dunk contest.
I thought this would be a good time to actually talk about randomized controlled trials, trial design, and some of the different approaches and developments in the space.
This is a long topic, so I’ll be splitting it up into a couple posts. Today will be focused on laying some basics: what randomized control trials are, the shortcomings, and some tips when reading studies.
Just as a caveat - statistics was literally my weakest subject in college (outside of Spanish, different story), so read everything with that in mind. But I did work at a clinical trials startup called TrialSpark so I’ve looked into this quite a bit.
What is a Randomized Control Trial (RCT) and why should we do them?
What are randomized controlled trials and why do people love them so much?
Randomized - Patients are sorted into treatment buckets at random.
Controlled - There is a control arm, or reference group, which we can compare the results to.
Trial - You have a hypothesis that needs to be proven or disproven on some dimension of performance.
To run a trial like this, you would want patients to be put into buckets at random. Neither the patient themselves nor the person running the trial should know who’s in what bucket. Also in an ideal world, every single other variable is controlled for (age, gender, lifestyle, etc.) and we have a massive sample size so that the distribution of those variables between the buckets is somewhat equal + flukes are reduced.
The reason we need randomness is because we want to prevent selection bias. We wouldn’t want doctors to put patients in the treatment arm just because they think the patient would respond better, or they like those patients more, or those patients are sicker and probably need the treatment more. Without randomization, you could put all the young and healthier people in one group, all the really old and sick people in the group with the new drug, and then it’ll look like the drug did super well even if the effect was actually tiny.
The reason we need a control is because we need to compare the new treatment against something to see how much better the new treatment actually is. How can we prove it’s better than the regular treatment if we have nothing to compare it to?

If you only had one group of COVID patients and the experimental intervention was “Watching The Big Bang Theory”, most people would probably see the disease resolve naturally from their immune system and you would conclude watching the show is a good intervention because we’re not comparing it to anything. Also you’d probably see selection bias in things like “age” and “number of brain cells”.
This was seemingly the crux of the entire debate. If your sample size is large enough without randomization, could you theoretically remove that bias the same way?

The reason something like this would probably not be true is because people that CHOOSE to go get treated are a self-selecting group. Not only would you need the data from all the people that have the disease that did NOT get treated, there would need to be an equally large number of people that were afflicted with the disease severely enough that they should have gotten treatment but chose not to. The characteristics of the group getting treated and group not getting treated would need to be the same, which they aren’t.
There’s actually an analogy in VC. Imagine you wanted to measure the performance of tech startups as a category. If you only chose startups that received VC funding, no matter how large that sample gets it will always be skewed because the companies managed to pass a certain quality bar in order to receive funding (in a non-Softbank world).
This is why randomized control trials have been considered the gold standard. They theoretically control for the most number of variables besides the treatment itself and reduce the most bias in the process. However, they are not without their own issues.
The issues with randomized controlled trials in the real world
Ideally, these randomized control trials would be the silver bullet to solve the world of clinical trials and experimentation. However, when applied practically to clinical trials there are lots of shortcomings.
Long and expensive
You may have heard about how clinical trials take a long time, are expensive, Nikhil wouldn’t shut up about them for a year, etc. Well it turns out it’s actually very difficult to get everything you need to run a good randomized control clinical trial. Finding and recruiting a lot of patients for a specific disease area is really, really difficult and trials themselves require a lot of coordination, especially as the size of the trial increases.
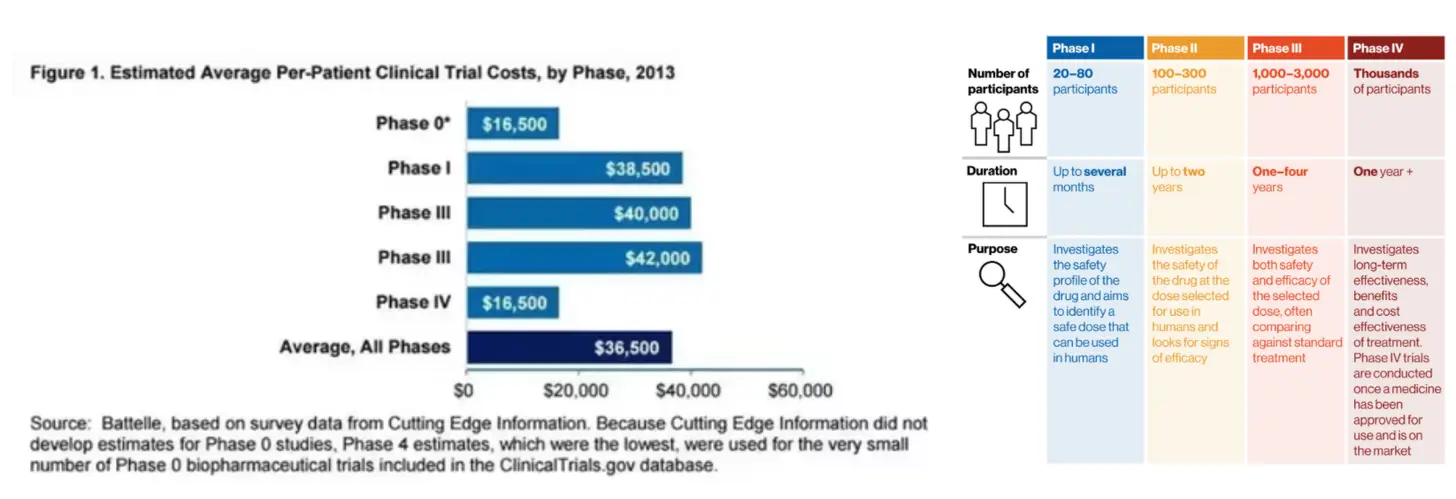
Selection bias (still)
Randomization can only help with selection bias after the patients join a trial, but there’s still a lot of selection bias when it comes to the kinds of people that choose to join trials in the first place. Many trials may not be physically accessible to patients because the trial sites are far away, or the recruiting tactics to bring patients into trials will skew to certain demographics (e.g. through online advertising), etc. The lack of racial diversity in clinical trials has been particularly top of mind in the clinical trials space.
Unrealistic conditions
Increasingly, we’re discovering an “efficacy-effectiveness gap”, where drug performance in a clinical trial perform vs. the real world is very different. A clinical trial is an extremely controlled environment, where you’re constantly monitored, a coordinator is following up with you, adherence to taking the medications is thorough, etc. Also the quality of treatment, facilities, and physicians at academic medical centers like NYU or the Cleveland Clinic where most clinical trials are best-in-class.
The real-world doesn’t look like that. You’ll have patients that don’t take the drugs when they aren’t followed up with, patients that have characteristics or underlying conditions that had low representation in the clinical trial, physicians that don’t prescribe the drug correctly or advise patients properly, etc.

End up being small and specific
A misunderstood issue to newcomers in clinical trials - patient recruitment is not just hard because patients don’t know trials exist as an option (though that’s partially true too). One big reason is because clinical trials are trying to control for as many variables as possible to show efficacy of their treatment by having VERY specific criteria for who they allow in a trial to reduce confounding variables + get people they think the drug will work particularly well for.
I went to clinicaltrials.gov and picked the first Phase II trial I saw, take a look at the inclusion/exclusion criteria. This is literally 10 of the 27 criteria that the trial is looking for.

The more variables that researchers try to control for, the harder it’s going to be to find patients that meet those criteria and the sample size will inevitably become smaller.
Poor at measuring the effect of non-controlled variables and long-term effects
The goal of a randomized controlled trial is to see if the specific intervention has a measurable change on a specific disease. But what if it turns out there was some other variable that actually did play a massive role in how someone responded to some medicine (e.g. your genetics)? It would actually be very difficult to ascertain that information if your trial design had not measured for it, even if that information is very important. This is especially true for any intervention that might change with lifestyle modifications, e.g. diet, exercise, etc.
Additionally, because most trials are trying to finish in the shortest amount of time possible, they typically don’t wait to see how some of these interventions impact the people in each group in the long-term and instead focus on the most immediate metric that would determine an intervention is successful.
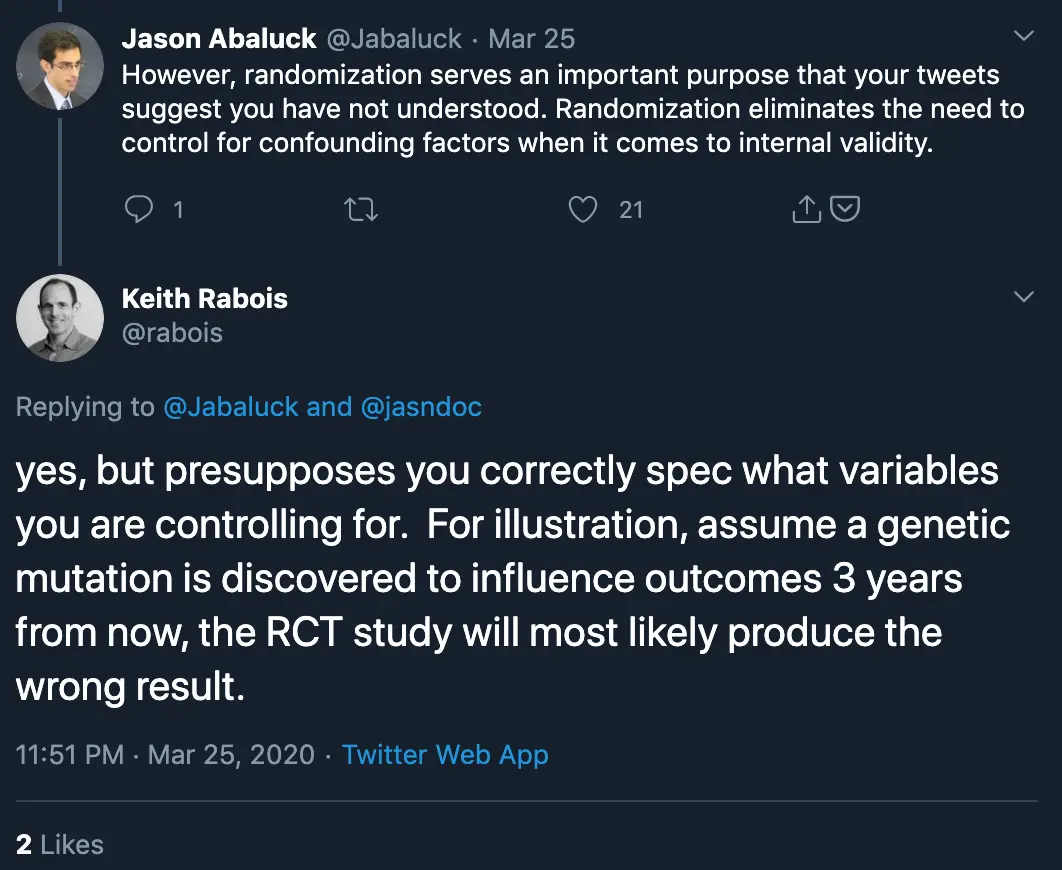
Keith’s point here is true. If there’s an unknown variable that impacts a study (especially one that requires a long period of time to discover), then a randomized controlled trial will not be able to test its influence well. It’s the reason why you want to get data from the real-world to complement the trial - to discover these new potential variables to consider, and possible force you to re-evaluate existing results or run new randomized controlled trials with that in mind. However, that doesn’t necessarily mean you should replace the randomized control trial in the first place.
Measuring what you want can be really difficult
Most randomized control trials are trying to find the effect on a disease, but what metric should you measure to determine if the intervention is successful? The metric you’re trying to improve is called an “endpoint”, and it’s actually very difficult to figure out which endpoint to pick to see if a drug is working. If a drug is trying to cure a disease, what measure are you going to use to prove a disease completely gone? If a drug is trying to slow the development of a disease or reduce symptoms, which measures make the most sense?
A particularly hot topic is the use of “surrogate endpoints”. In many cases but especially in cancer, the true endpoint we want to measure is survival rate. However, that would cause the trial to drag-on for an extremely long time. So we look for proxies (e.g. tumor shrinkage) that can give us some information on whether the drug is working in a shorter period. But there’s a lot of beef about which surrogate endpoints are actually good, if we should be using them, etc. Diss tracks coming soon.

Ethical?
Critics of randomized control trials might talk about how putting people on placebos or denying people new treatments that are clearly very effective is unethical. I do want to clarify a few misconceptions about this one.
- There are lots groups (IRBs, ethics committees, etc.) and ethical guidelines (GCP/ICH) to make sure that studies are designed ethically. This stuff isn’t done secretly.
- Part of adhering to these ethical guidelines is understanding when a placebo is the right move for the control arm. If there is a drug/intervention that works really well for a disease and the mortality rate without that intervention is very high, it would be unethical to use a placebo. Instead new therapies would be compared against the regular treatment (aka. standard of care). For example, chemotherapy works really well against testicular cancer, so the control arm for a new testicular cancer drug would likely be chemotherapy. Not every trial is going to put you on a sugar pill, especially in life threatening cases.
- Biopharma companies know that if people thought they were going to be randomized to the sugar pill group, they would be less likely to enroll in a trial or complete it. So most biopharma companies weigh the chance of not enrolling patients vs. having a true control group with only a placebo.

Reading Studies
My favorite joke study of all time is about a randomized control trial that had people jump out of airplanes with or without a parachute. The whole thing is worth a read and good satire about the religious adherence to randomized control trials and how poor study design can mislead results.
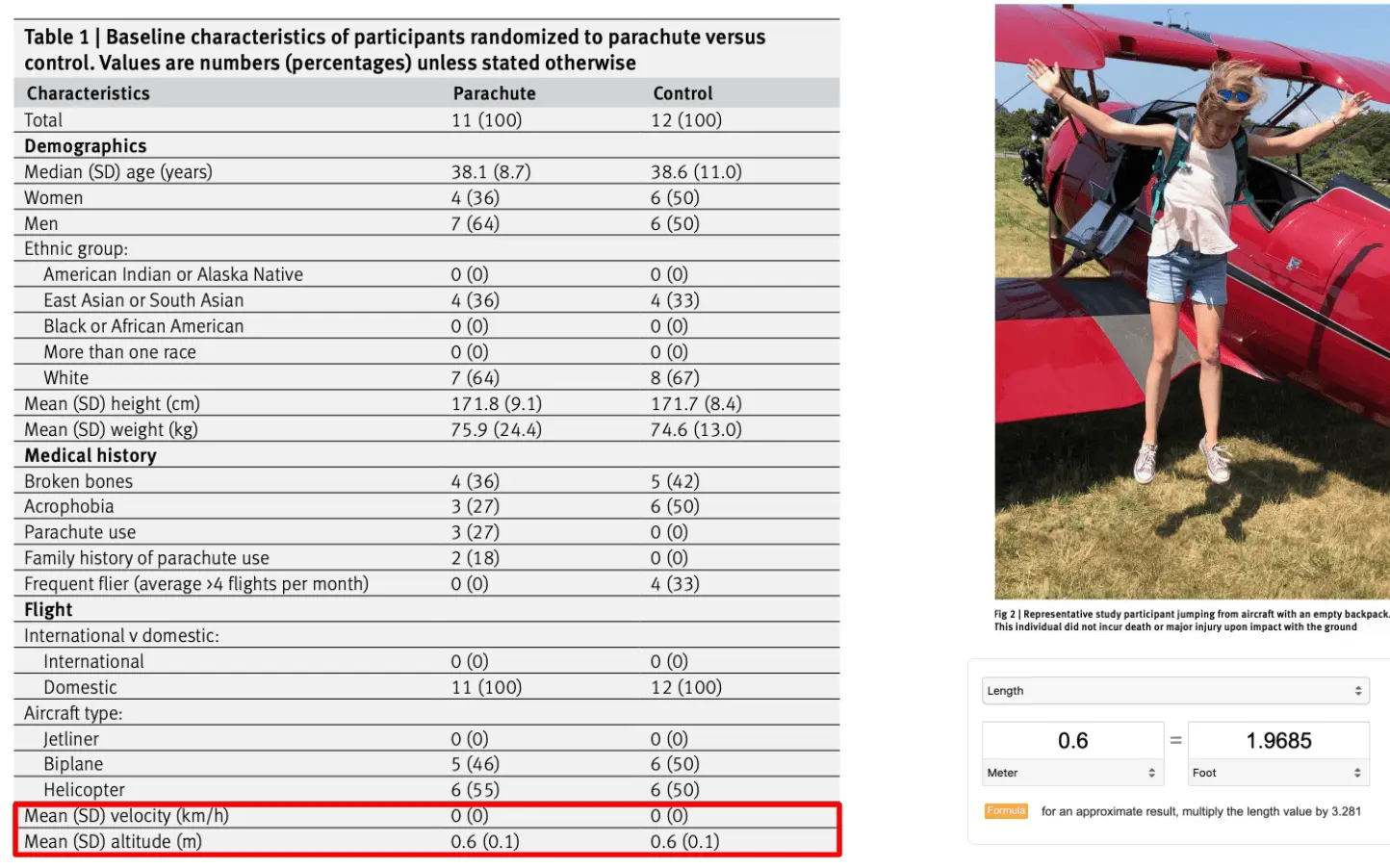
We have performed the first randomized clinical trial evaluating the efficacy of parachutes for preventing death or major traumatic injury among individuals jumping from aircraft. Our groundbreaking study found no statistically significant difference in the primary outcome between the treatment and control arms. Our findings should give momentary pause to experts who advocate for routine use of parachutes for jumps from aircraft in recreational or military settings.
Because there have been so many papers floating around now related to cures for the pandemic, some things to consider when you’re looking at the results of a study to see if it’s legit.
- How many groups are in the study? Is there a control group? Who constitutes the control group? How many people are in each group? Are the patients all at the same level of severity or cycle of the disease? You don’t want to compare a person with COVID in critical care with someone that just started displaying mild symptoms.
- Where is this study recruiting patients from and what is the screening criteria? Does that skew a certain way? Are the people in the study homogenous?
- How many people finished the study? Why did people drop out or get excluded from the study/results?
- What was the efficacy of the treatment and was it significant? How significant? What was the variance in that efficacy amongst the participants?
- Compared to the regular treatment that would be used, how much better is it? How do the side effects compare? Is one easier to take, or better for some specific type of patient?
- How do these results compare to other papers that might be looking at the same drug or have similar trial designs? Is it starkly different? What might explain that?
I’m probably missing a lot of questions but these are some to think about. For example, one paper that was circulating widely about hydroxychloroquine use in COVID patients had this in its results section:
A total of 26 patients received hydroxychloroquine and 16 were control patients. Six hydroxychloroquine-treated patients were lost in follow-up during the survey because of early cessation of treatment. Reasons are as follows: three patients were transferred to intensive care unit, including one transferred on day2 post-inclusion who was PCR-positive on day1, one transferred on day3 post-inclusion who was PCR-positive on days1-2 and one transferred on day4 post-inclusion who was PCR-positive on day1 and day3; one patient died on day3 post inclusion and was PCR-negative on day2;
Out of an arm that had only 26 people, they straight up excluded 3 people because they ended up in the ICU and 1 one because they DIED.
Like…you can’t just exclude them lol.
Next time on Out-Of-Pocket
Designing trials is not some cut and dry thing - there is no definitively “right” way to do a trial. The choice of trial design and when to do a trial assesses the tradeoffs of speed, statistical bias, cost, side effects of the potential therapies, and impact to patients (both that are on the trial and who would benefit from a cure).
Personally? I still think randomized control trials are our best tool to run a true experiment with the least amount of statistical bias. But again, I’m not a stats god. I do genuinely think there are situations like pandemics where we need to rethink the risk-reward ratio of running a full-blown randomized control trial. We might come to the same conclusion that we do in fact need them, but I think it’s important to have the discussions about why without shitting on each other. These are weird times, we need to be open-minded about finding solutions while also realizing that maybe we run randomized control trials for a reason.
In fact, I think the below tweet is effectively what Keith was trying to say all along. RCTs are not realistic for CERTAIN situations, and so we have to rely on other types of data (including data from the real world) in place of them because we have no choice.

In the next issue, I’ll talk about real-world evidence (which Keith was referring to) and their limits, interesting clinical trial designs, and where clinical trials seem to be heading.

Thinkboi out,
Nikhil aka. “Sufficiently Large N”
If this was forwarded to you, you should sign up! Please. Don’t make me beg.
Interlude - Courses!!!
See All Courses →We have many courses currently enrolling. As always, hit us up for group deals or custom stuff or just to talk cause we’re all lonely on this big blue planet.